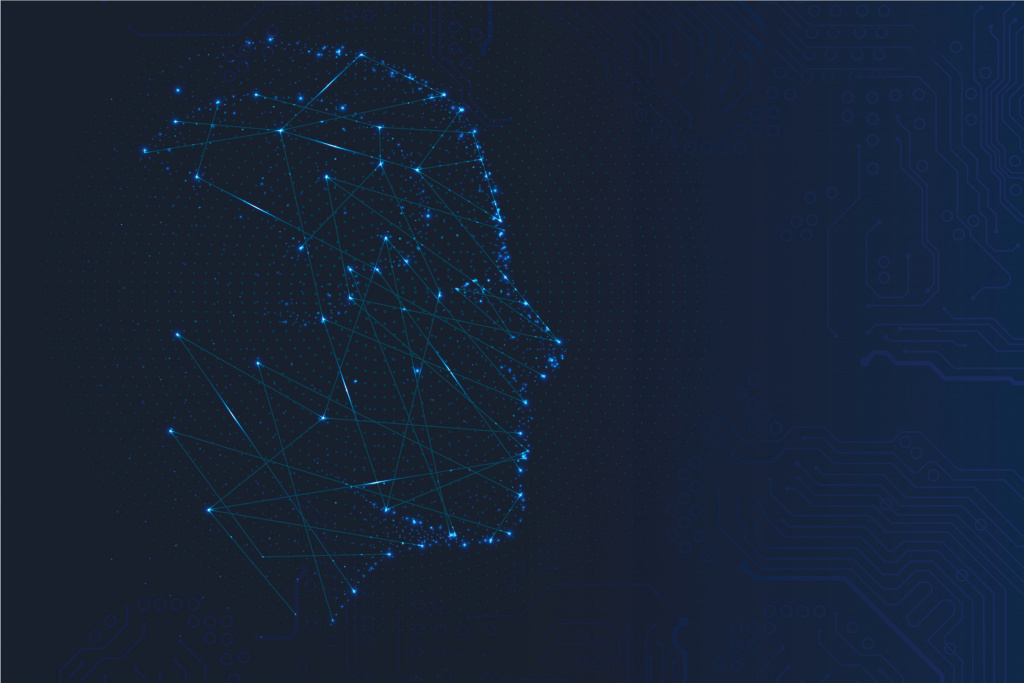
In the age of algorithms and artificial intelligence (AI), the number of adaptive and personalised learning education products vying for educators’ attention and funds is immense. Using the latest advances in machine learning, big data and learning analytics, adaptive and personalised learning products can process a range of data, (educational, psychometric and social media), to map individuals quantitatively, compare them globally and generate recommendations for their learning potential and pathways. These techniques are being translated into impressive learning gains in the context of standardised curricula.
For example, LEAP Innovations, a Chicago-based research and piloting company found a 13 per cent increase in literacy using an AI-based reading product versus the control group. Laudatory testimonials from schools featured on product websites means it is easy to slip into a ‘black box’ mentality, focusing on the application of these technologies, without further unpacking the mechanics of how they produce the outcomes. However, by understanding the mechanics behind the production of profound learning insights and results that these products offer, educators can develop personalised learning strategies that make the most of the strengths and limitations of AI.
Opening the black-box: Artificial, adaptive and personalised learning
Knowing which questions to ask relies on understanding how these products are designed. AI, adaptive and personalised learning are the latest education technology buzzwords, but what distinguishes them? While there are many types of AI, they all involve some form of learning and applying knowledge to achieve a purpose. Learning and problem-solving is something that humans do very well, but sophisticated AI systems, along with huge data sets and computing power, can process and compute complex problems at a scale unfeasible for humans. AI systems can learn autonomously, which means we do not have to invest time and resources into writing code ourselves. A classic example of AI in education technology products is adaptive learning, which refers to the data analytics strategies that algorithms use to continuously learn about how individual students learn, and thus optimise learning sequences.1
Adaptive Learning
As students use adaptive learning software, they generate countless data points, which are recorded and fed to algorithms. These algorithms have been designed to learn from this data to better perform against designed criteria. An advance in a type of machine learning, using neural networks, has accelerated the functionality and potential of machine learning. Artificial neural networks are statistical models that are based on the way that the brain computes and processes information in non-linear, continuous and adaptive ways. Deep learning occurs when these artificial neural networks and learning algorithms observe and analyse raw data, learn, identify patterns and make predictions to determine optimum outputs.
Algorithm-based personalised learning
In the case of the adaptive learning education technology products, such as ALEKS and Dreambox, these algorithms have been designed to identify the ideal learning pathways for an individual student based on the criteria derived from neuroscience and cognitive-based learning and memory theories. These theories emphasise the importance of reinforcement and retrieval in short and long-term memory, and how this can be improved through interleaved and spaced learning and assessment. Every student’s ideal pattern of interleaved and spaced learning, forgetting, retrieval and reinforcement will be different. The algorithm’s or ‘machine’s’ capability of operating autonomously and writing its own code means it can learn how the student learns, what they have mastered and which areas they require improvement in. These insights are used to diagnose gaps in knowledge and skills, make predictions of the ideal learning pathways that will fill these gaps, and translate these into personalised recommendations. With the current computing power available, such inferences can be made in nanoseconds. The algorithms learn from data, and continuously teach themselves to get better. Thus, the more a student uses such software, the more data there is generated, the more the machine learns and the better it gets in making predictions and determining the ideal sequence of interleaved learning and assessment, i.e. personalising learning. Put simply, machine learning provides personalised learning for mastery.
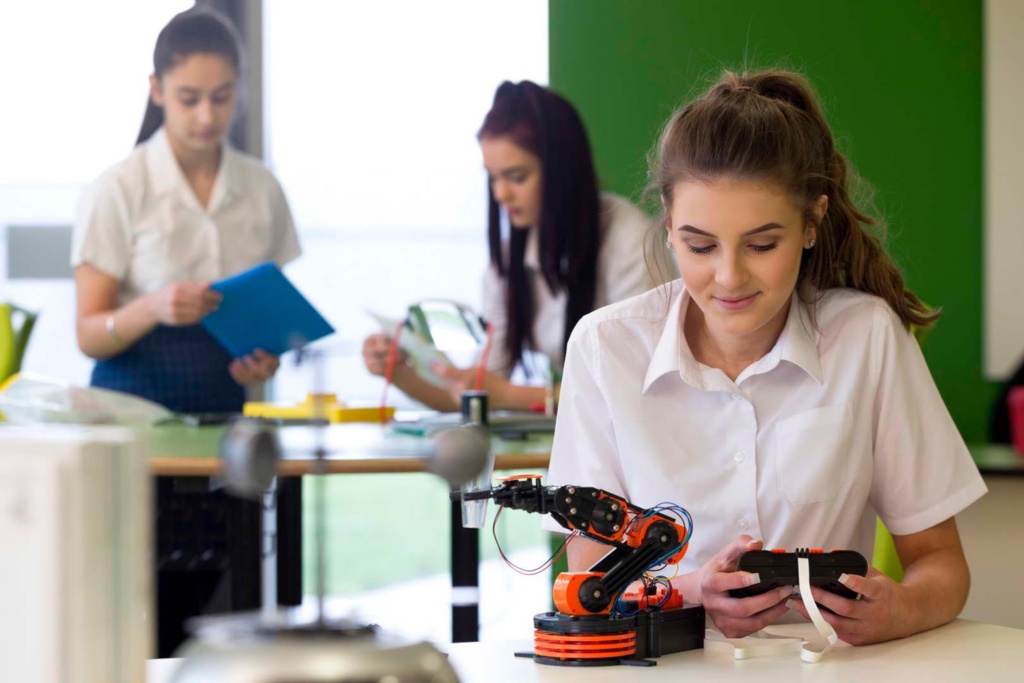
More than personalised learning pathways
It is not just pace that can be personalised—more sophisticated products personalise according to learning preferences, complexity of tasks, students’ interests, and special needs. A few products enable educators to customise systems through gamification, using simulations and apps. Many products provide smart content services, using AI to process textbooks and resources into learning pathways and assessments for students, effectively designing digital courses for educators. For leaders and those desiring a macro perspective, some products offer aggregated analytics at the class, cohort and national level.